The COVID-19 infection fatality rate (IFR) is the proportion of people infected with the virus that die. It’s a simple ratio of deaths to infections that has been surprisingly difficult to pin down. It’s also a key to understanding the impact of the pandemic and narrowing down estimates for its future course.
Early modelling by Verity et al. (2020) pointed to a 0.66% IFR in China, pointing out that IFR increases with age. This result was useful in early modelling of the pandemic, but adjustment was required for countries with different age profiles, with younger populations expected to have a lower IFR and vice versa.
Researchers estimate the IFR using data on COVID deaths and representative antibody seroprevalence studies. These seroprevalence studies estimate the number of people in a population that have antibodies and, with some allowance for the sensitivity of these antibody tests, can be used to estimate the number of infections in that population. The ratio of COVID deaths to infections in that population can then be estimated at both a general and age-specific level. Note that case numbers are not a reliable measure of infections as many people never get tested.
Levin et al. (2020) provided a meta-analysis of such research. They combined estimates from many studies to come up with estimates of the IFR by age. Work like this has allowed modellers to estimate how many people have been infected by working backwards from the COVID deaths using the IFR.
These studies require representative seroprevalence data as well as an accurate measure of COVID-19 deaths, both of which are difficult to come by in developed countries and even harder to obtain in developing countries.
The COVID-19 Actuaries Response Group has shown that COVID deaths were underreported in developed countries such as the UK, especially early on in the pandemic. We’ve also shown that developing countries such as South Africa and many around the world are underestimating the true impact of COVID-19 on mortality. Seroprevalence studies are also more difficult to run in developing countries.
Estimates have been made in smaller settings in developing countries or using potentially biased data. As an example, the preprint by Mhlanga et al. (2021) estimated the IFR in South Africa using seroprevalence estimates from antibody testing of blood donors, and excess deaths. It is not clear how representative the blood donors are of the general population.
In a new preprint, Levin et al. (2021) have pulled together various sources of data from around the world and combined these into IFR estimates for the developing and developed world. Their approach was to:
- Identify seroprevalence studies that were done using representative samples.
- Make an allowance for sample uncertainty, as well as the sensitivity and specificity of the testing assays used in these studies
- Allow for seroreversion over time. This is the phenomenon that over the months following infection, fewer and fewer people will test positive for antibodies.
- Use suspected and confirmed deaths, where available, to estimate IFRs. They had to vary their approach to try and allow for underreporting of COVID deaths.
Results are shown below. The graph is on a log-scale so straight lines represent exponentially increasing IFR by age. One can see that the estimate of the IFR in developing countries (red line) is higher than the high-income country benchmark (green line).
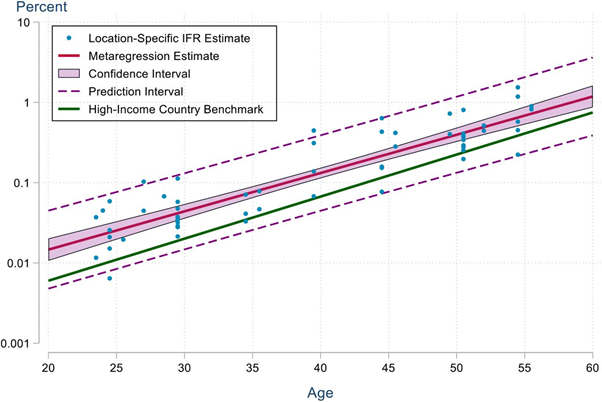
The authors findings are that:
- Seroprevalence in developing countries was higher than in developed countries, indicating more people had been infected.
- Seroprevalence was similar across age groups in developing countries. In developed countries older age groups tend to have lower seroprevalence. This indicates that in developing countries the elderly were less able to avoid getting infected compared to their counterparts in developed countries.
- Even though average IFRs may be similar or lower in developing countries, the age-specific IFRs were between 1.3x and 2.5x higher in developing countries compared to developed countries.
- The difference is higher at the younger ages than at the older ages, indicating that in developing countries the severity of the disease is higher for younger people.
- They conclude that the burden of COVID-19 is far higher in developing countries due to increased transmission amongst the elderly, as well as to higher age-specific IFRs which may reflect lower access to health care.
The results of this meta-analysis are consistent with mortality experience emerging in South Africa, both in the general population as well as life insurance data. The observed excess deaths in these contexts, combined with results from the limited seroprevalence studies that are available, are consistent with higher age-specific IFRs.
The data used in the new estimates predates the Delta variant. Emerging research from Public Health England, Sheikh et al. (2021) and Fisman et al. (2021), point to Delta having more severe outcomes (hence probably a higher IFR) than variants that preceded it. Those variants may have already had more severe outcomes than the original wild-type strain.
It is clear that speaking of “the IFR” of COVID-19 is taking a simplistic view and that various factors drive the severity of COVID-19 in a particular setting.
The implications for understanding the impact of COVID-19 in developing countries such as a South Africa are:
- IFRs derived from data in developed countries require adjustment to be appropriate in the developing world.
- IFRs at younger ages, which form a bigger part of the population in developing countries, are higher than for developed countries.
- Where lower IFRs were being used to estimate the proportion of a country infected, those estimates need to be revised downwards (as a higher IFR implies lower number infected to date).
- A higher IFR needs to be used in projections of future pandemic impacts. This may be even higher than estimates derived in the meta-analysis described above because of the emergence of Delta.
Increasing IFR assumptions has a gearing effect on projections of future mortality in pandemic. Increasing the IFR reduces estimates of infections to date, and thus increases the scope for future infections. Those future infections will then result in a higher mortality burden. New, more virulent variants, further worsen this situation.
There are also implications for vaccine distribution. In a previous bulletin it was shown that “excess vaccines may save potentially 11 times the number of lives in a low-income country that has not administered any 1st doses as yet compared to being used as a booster in a high-income country.”
The simple model used in that bulletin made no allowance for a higher IFR in the low-income country. We pointed out in the bulletin that it was likely to be higher and therefore 11x was likely to be an underestimate. As Levin et al. puts it:
“These results underscore the critical need to accelerate the provision of vaccine doses to vulnerable populations in developing countries.”